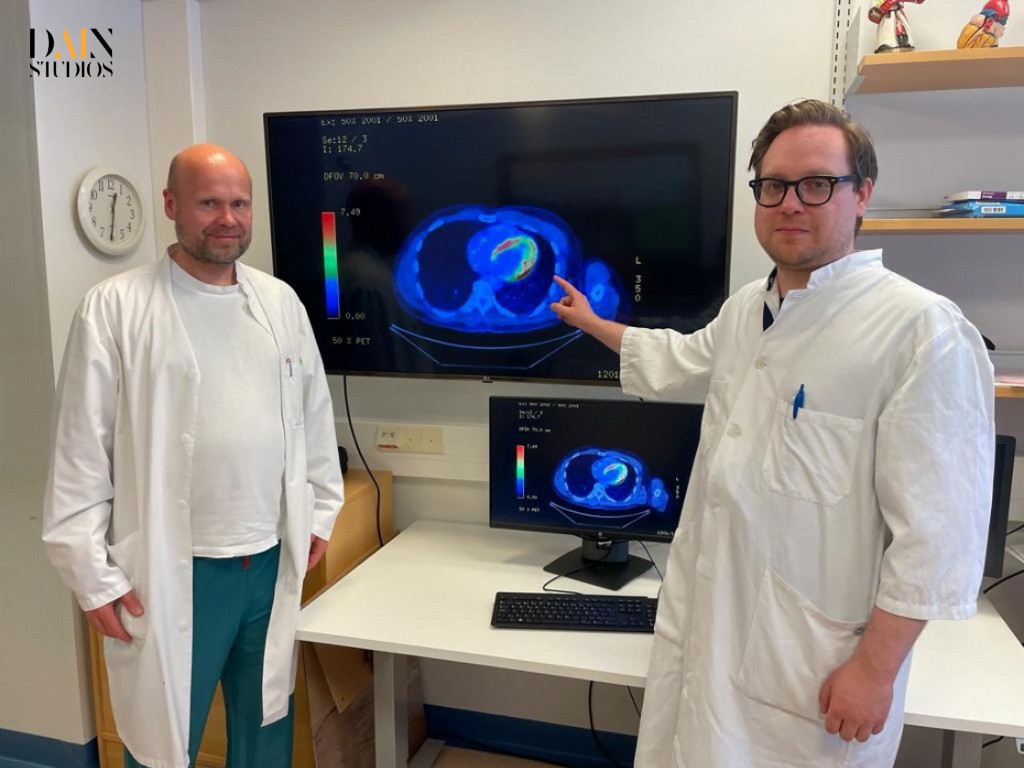
AI & Positron Emission Tomography (PET)
“See that yellow arc-like intensity?” says Valtteri Uusitalo, a cardiologist at Helsinki University Hospital (HUS), pointing to a row of glowing images on a computer monitor. “That’s where the artificial intelligence (AI) model is focusing on the 3D PET-scan of the heart.” Positron Emission Tomography (PET) is an imaging technique that uses radioactive tracers to detect high rates of metabolic activity. Abnormal activity is often a tell-tale sign of disease. In this case, the AI model is telling Valtteri Uusitalo and his colleague, Jukka Lehtonen, that it is very likely a case of cardiac sarcoidosis, a rare and often fatal inflammation in the heart which even the most experienced specialists have a tough time identifying.
The heat maps at the bottom of the cardiologists’ screen are the result of a year’s work with colleagues at HUS and data scientists at DAIN Studios – an AI consultancy with offices in Helsinki, Berlin and Vienna. They flag the suspect tissue in the heart from the front, the side and the bottom, combining CT (x-ray computed tomography) scans of the heart’s contours – shown at the top of the screen – with the PET images of metabolic activity shown in the middle, and the areas that have drawn the AI model’s attention (see Figure 1).
“The AI can see patterns in the images which the human eye cannot...This is of huge practical importance for medicine.”
Jukka Lehtonen
Having been trained on an archive of combined PET/CT scans and diagnoses of hundreds of former HUS patients, DAIN’s software is currently over 93 percent accurate in identifying cardiac sarcoidosis – so-called ‘true positive’ result or, in its absence, so-called ‘true negatives.’ “That’s as good as a clinician,” says Ulla Kruhse-Lehtonen, one of DAIN Studios’ co-founders and CEO of the consultancy’s Finnish operations. “And with more data, it will start to do better.”
After training, validating and testing the software on a dataset from HUS, the next step will be to test it on data from elsewhere – talks with hospitals in the USA and Japan are underway.
A ‘Superpower AI?’
"This is the kind of ‘superpower AI’ that will make important contributions to our lives – if we use it correctly...This AI does not decide for itself, it is strictly an assistant to the doctor in charge, and it is what we call explainable AI – it ‘explains’ it's diagnoses by showing what it sees or doesn’t see on the heat maps.”
Saara Hyvönen
You can see this when comparing Figure 1 (b) – PET intensity – to (c) – heat map, which shows the areas where the model has focused in making it decision.
Dirk Hofmann, a fellow DAIN co-founder and CEO of its German business, says: “Our vision is for this software to become part of medical-imaging machines, so that doctors not expert in cardiac sarcoidosis can still diagnose such a rare disease with confidence.”
The promise of improving medical diagnoses and treatments, increasing the efficiency of clinicians and optimizing the allocation of resources has led to an increasing buzz about AI-assisted healthcare. The research firm Verified Market Research, for example, predicted that global sales of AI-powered diagnostics solutions will rise fourteen-fold, to USD 10 billion between 2021 and 2030.
DAIN’s approach is to harness the upsides of AI, while putting explainable AI to use in order to address the potential clinical, social and ethical downsides, such as possible errors and patient harm, possible decision biases, and lack of transparency and trust.
Explainable AI (XAI) is a way to keep a vigilant human eye on the work of the mathematical model driving any AI-application – an opportunity to follow its reasoning, or if need be, to turn it off. In 2021-22, DAIN took part in a Business Finland funded program called AIGA, short for Artificial Intelligence Governance and Auditing, which has the self-declared aim of “developing solutions for putting responsible AI into practice.”
Hugo Gävert, DAIN’s Chief Data and AI Officer, recalls: “AI governance and auditing is crucial in the field of medical imaging, and we were looking for ways to bring explainable AI to the medical domain.”
Revolutionizing diagnostic procedures using AI
Down the road at Helsinki University Hospital, Lehtonen, Uusitalo, and their colleagues were engaged in what Lehtonen calls their ‘old struggle’ of diagnosing patients with cardiac sarcoidosis: the telltale patterns of unusual metabolic activity in the heart were difficult even for the most experienced specialist to spot on the best PET scans; and the only way of definitively knowing whether suspected cases were true or false alarms was by conducting a tricky needle-biopsy of the heart tissue.
“The procedure often has to be repeated,” Lehtonen says. “It’s a long and frustrating road to diagnosis. It usually takes weeks – and I’ve seen it take six months.”
The team at HUS had already investigated ways of shortening this wait. They had, for example, unsuccessfully explored quicker approaches based on RNA sequencing. “I guess AI was the next logical step,” Lehtonen remembers. By chance, HUS had over one thousand PET scans of suspected cardiac sarcoidosis cases – and their eventual diagnoses – because a respected cardiologist at the hospital had for over a decade compiled a huge imaging data set of these cases. “The Finnish material is remarkable,” Lehtonen says. “However, I still didn’t have high hopes.”
But when doctors then met the data scientists, things quickly changed.
“Their cardiac sarcoidosis data was unparalleled...This was a huge trove of imaging data and biopsy-verified diagnoses – we’ve since looked into this and nothing of this size exists anywhere else in the world.”
Hugo Gävert
The quality of the 3D scans was good enough to train deep-learning algorithms to discern links between the patterns on the PET and CT images and the eventual diagnosis based on laboratory examination of the biopsied tissue. “We knew we could build and train an AI model for that job.”
DAIN’s data scientists first anonymized the data from nearly eight hundred cases to protect patient privacy. Before training the AI model, they preprocessed the images, visually isolating the heart in both CT and PET scans. “Segmenting the heart is a challenge as its borders are not always clear in the CT scans,” says Gävert. “But this step is essential as nearby lymph nodes can also show high activity in the PET images.” Gävert and his colleagues then split the data set into training, validation and test sets, a standard technique in AI to make sure different data gets used at every stage.
The preprocessed images were fed into a self-learning neural network, popular for image processing.
We used what’s called a residual neural network, which can be built to pick out and categorize ever more complicated patterns...The deeper the network, the more sophisticated the objects it can identify.”
Silvio Jurk
To this, DAIN added a technique called attention-based modeling so that the software would not only tell users whether it computed an image to be a positive or negative case – it would also show them what patterns in the image made it decide this.
Troubleshooting and tweaking the model
Between June and November 2022, the DAIN team tested and tweaked the model, so that it would catch as many as possible of the test-sample’s cases identified by biopsy. “In the end, we missed very few of the true positives – and that may have been because of a problem with the original data,” says Garold Murdachaew, who took the lead in testing the model at DAIN. By design, the model is currently less good at identifying true negatives.
“We deliberately still over-diagnose cardiac sarcoidosis – doing a biopsy to gain certainty that someone is not ill is less costly in human terms than missing a positive diagnosis altogether.”
Garold Murdachaew
Over at HUS, Lehtonen supposes that if the software does as well with other datasets as it did with the Finnish one and then jumps through all the regulatory hoops, it could in three years time be in clinical use to at least rule out cardiac sarcoidosis. “For many people, it could potentially cut the diagnostic delay to days or weeks rather than the current weeks or months.”
He does not see AI replacing the scientific certainty of the biopsy any time soon – although that, of course, is the ultimate goal. “The system is easy to use and understand,” he says. “We now need to gather experience with it, but it does look very promising.”