Any large scale transformation starts with establishing a vision and strategy. According to a recent survey, 99% of Fortune1000 companies reported investments in data & analytics with less than 30% of them claiming to have achieved transformational impact. Coincidently, just 30% of them have developed a well-articulated data strategy. While these numbers are not 1-to-1, they underline our experience that many data & AI transformations fail to break the barrier of success due to a lack of strategic vision, leadership support and a strong focus on value generation: what we call the impact drivers.
This is Part 2 of our four-part article series explaining how we approach and advise our clients on their data & AI transformation journeys, introducing the DAIN Data & AI Maturity Model.
- Part 1 gives an overview the DAIN Data & AI Maturity Model (DAMM).
- Part 2 (this article) dives deeper into the strategic impact drivers.
- Part 3 details the organisational and human enablers.
- Part 4 gets to the core technologies of data & AI transformations.
Let’s start from the top
The DAIN Data & AI Maturity Model divides the elements into two main categories; Impact drivers and Enablers. The impact drivers ensure overall strategic support and consistent focus on value delivery. The enablers are the underlying organizational and technological capabilities fundamental to delivering and scaling data & AI use cases.
This article focuses on the two impact drivers; Strategy & Vision and Use Cases & Value Generation, and the first enabler; Leadership. These elements set a direction for the organization, and have a big impact on how mature the rest of the enablers need to be to achieve the common goals. Therefore, an organization should ideally first define and communicate these impact drivers, before setting the whole organization to work on data use cases.
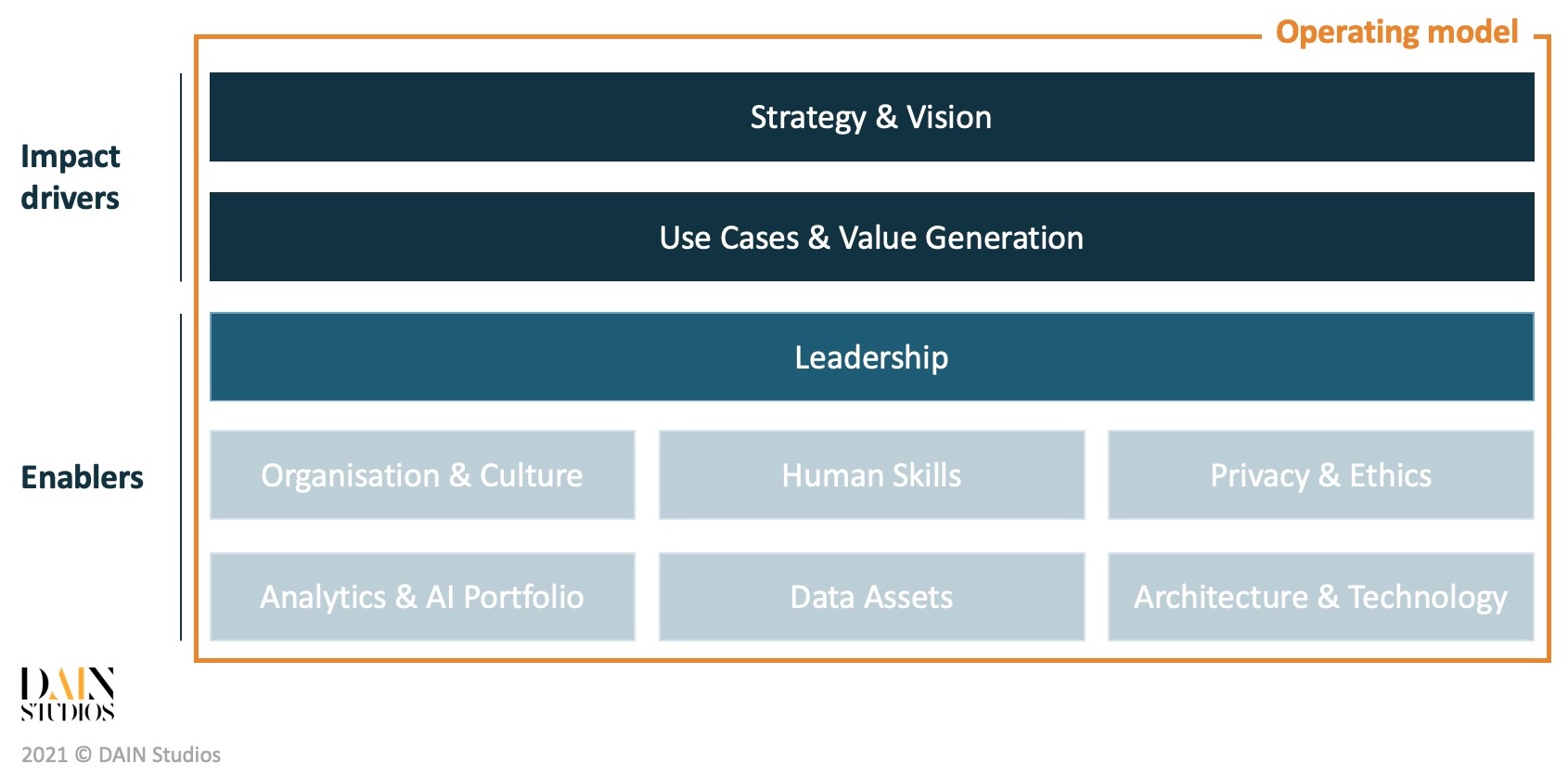
Strategy & Vision
A significant part of business strategy today is digital transformation. In short, it means the integration of digital technology into all areas of business. Since data is crucial to many digital initiatives, digital transformation will only work when it is equipped with the right data.
For making sure the data & AI development is aligned with the business objectives, organizations nowadays commonly create a data strategy. Data strategy is a subset of or subordinate to the company strategy. Its purpose is to make sure that stakeholders have a common, long-term direction and goals for using data & AI. Thus the data strategy helps the organization move forward with the various enablers that need to be developed to support these goals. Enablers are organizational skills or technological capabilities that are needed to support the overall data & AI maturity of an organization.
Companies in their data & AI discovery phase typically do not have a data strategy, or it is not officially documented. One of the first steps you can take is to understand what opportunities others in your industry are already taking advantage of. Based on this, you can formulate your own shared vision for the use of data and align this vision with the overall strategic objectives of the company. Some of the leading organizations, when it comes to the application of data & AI, already have their data strategy strongly integrated with their overall strategy, to the extent that data and analytics in all organizational functions are considered as the norm.
One public utility company we advise asked us to aid with their demand process and prioritization of data use cases. During the project it became clear that relatively good processes were already being implemented. A real issue was the disconnect between the management and the data & analytics team. A major gap was the lack of clear vision where the organization aims with investments into data. The data and analytics team didn’t know where to focus, and the management didn’t receive the business value that they were expecting. The obvious solution was to start from setting a common vision for the use of data.
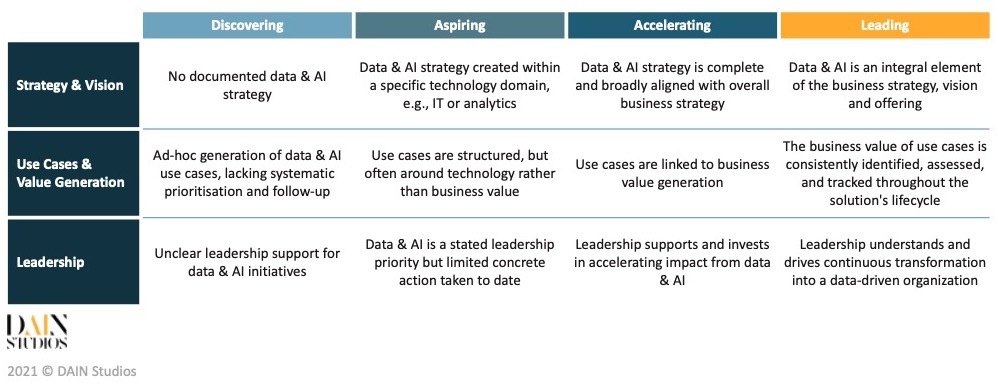
Use Cases & Value Generation
The second impact driver is Use Cases & Value Generation. This element highlights how use cases are generated, prioritized and how business value generation is ensured, when it comes to applying data & AI in your company.
Commonly the use of data in an organization starts by sponsoring ad-hoc projects or pilots, possibly executed by external vendors. The business goals are often too high level and non-actionable, the outcomes of the pilots are not carefully monitored and the results are commonly forgotten when the project ends. These kinds of pilots don’t usually generate a lot of direct business impact and are difficult to replicate. The collected value from these experiences is more indirect – understanding what could be achieved with data if you were to invest into it. To aid with use case development, we have implemented an Analytics Playbook with several of our clients successfully. The Analytics Playbook is a set of guidelines and instructions created together with clients that document how to build data use cases in practice within the context of a specific organization.
Consider the case of a Nordic retail chain that wanted to use AI to detect delays in its supply chain. A detailed analysis was delivered to the business stakeholders explaining the top five reasons why the deliveries were occasionally delayed. The supply chain managers indicated that they were already aware of these reasons, as they had manually analyzed the data over many decades. A more structured approach to developing the use case idea and value hypothesis, considering what was already known to the organization would have allowed the project to skip directly into solving the ‘unknown’ issues, delivering impact earlier without hindering the otherwise large investments in data & AI capabilities.
For an organization to elevate its data & AI maturity, the business value in a use case needs to be identified, designed and monitored from ideation through delivery, all the way to production and operation. The outcome of more careful use case design is the ability to have more direct business value at the end of the project. And as a side effect, the delivery usually becomes faster, as the team has a better understanding of the expectations and objectives from business stakeholders.
Lastly, the ability to integrate the results into business processes is paramount to generating value from data & AI. This may sound like an obvious step, but all too often we see project results ignored under the excitement for the technology itself. Data use cases create business value by improving, optimizing or changing business processes. To make that change permanent and effective requires adaptation in the way people make decisions and take action based on the newly found insights and predictions, i.e. you need to ensure the data is guiding the processes going forward.
Leadership
Ensuring impact on your data & AI journey is difficult without leadership support. Therefore, Leadership is conceptually on the top of the other enablers as it is an essential motivating factor for developing data capabilities, although tangible work mostly happens in the operational levels of the organization.
The first indicator for leadership support is simply the commitment, where the management recognizes and communicates the importance of data. This can start from openly recognizing data as a strategic topic and continue to a situation where one or all executive team members have responsibility for data & AI initiatives.
Data transformation requires big changes, new roles to be introduced and skills to learn. It will not happen automatically by just placing it as a strategic objective. The teams working on the initiatives will face conflicts of interest, trade-offs and lack of direction. Therefore it is critical that the leadership is involved and continuously supports the transformation.
The second aspect of leadership is assessing the ownership of the data & AI transformation. Without a defined ownership, data initiatives tend to “just happen” without alignment with other initiatives. One possibility is to assign an executive sponsor to data & AI transformation, which ensures that the topic is permanently on the leadership agenda.
Finally, improving data maturity requires adequate financial support from the organization to ensure the other enablers can be developed. Especially in the early stages of data transformation it is important to have a central budget available for building first enablers and capabilities. In our experience that speeds up the start significantly, as it avoids lengthy budgeting discussions.
We often consult our clients on the importance of leadership commitment and support to data & AI initiatives. What is commonly forgotten is that this is equally a journey for the leadership, just as much as for the organization. Initial excitement is welcome, but you need to ensure that the data culture sticks among the executives. This can require behavioral changes too, as being data-driven cannot be a one-off initiative. You can hold a keynote presentation on the importance of AI for your organization, but the real change will come from truly understanding, re-emphasising and playing the role of a data-driven executive every day of the week, 365 days a year.
How to prepare for the journey?
Data transformation is often not a walk in the park. The road can be rough and longer than initially planned. To make sure you are making progress, it is recommended to do the data maturity assessment repeatedly, continuously understand where you are on the path, and ensure no enablers are falling behind. To learn more about the journey it is recommended also to read a guide written by two of our founders: How to define and execute your Data and AI strategy.