In today’s rapidly evolving landscape, where data and artificial intelligence (AI) drive innovation and competitiveness, the ability to adapt and acquire new data and AI competences is crucial. As organizations embark on their data and AI transformations, equipping employees with the necessary knowledge and competencies becomes a strategic imperative. However, navigating the vast array of training options and determining the most effective strategies for skill development can be daunting.
It is important to recognize that this journey doesn’t happen overnight and there is no quick fix. Gaining new data and AI competences and keeping up-to-date is a continuous learning process that demands dedication and investment from both sides, the employer and the employees. For this reason, many organizations are eager to upskill as many people as possible as quickly as possible, leading to untargeted initiatives that usually do not yield the desired results.
By adopting a systematic approach, organizations can ensure that their training initiatives are purposeful, structured, and aligned with strategic objectives. This approach enables organizations to conduct comprehensive skills assessments, identify specific learning needs, and design tailored training programs. Moreover, a systematic approach allows for the measurement and evaluation of training outcomes, enabling organizations to track progress, identify areas for improvement, and demonstrate the impact of training on individual performance and organizational success, remaining competitive in today’s dynamic business environment.
The key to impactful data and AI literacy trainings
Data and AI re- and upskilling initiatives are complex change management endeavors that necessitate more than mere training of certain technological knowledge; they demand the cultivation of a conducive learning culture, a data and AI literate mindset, and a change in behaviors. Achieving this radical shift is no easy task. Despite the increasing emphasis on training data and AI literacy within organizations’ data strategies (2021, Gartner, A Data and Analytics Leader’s Guide to Data Literacy), many struggle to derive tangible business value from these initiatives (2019, HBR, Where Companies Go Wrong with Learning and Development ).
Common pitfalls in data and AI literacy programs often stem from a lack of alignment with the business strategy and data and AI goals. When training initiatives are not closely tied to the organization’s overarching objectives, they may fail to deliver tangible value and relevance and lead to unclear learning goals of initiatives. This uncertainty usually results in insufficient commitment by leadership and inadequate allocation of resources, such as funding, time, and access to technology and expertise needed to design impactful learning solutions. Furthermore, the lack of direction leads to an “one-fits-all” approach, trying to teach everybody the same content while overlooking the diverse job specifics and individual needs within the organization. Such generic approaches limit the program’s ability to meet the diverse learning needs of participants, negatively impacting the engagement and knowledge retention.
To overcome these pitfalls, organizations should focus on three dimensions when designing training initiatives: Learning Objectives, Learning Environment, and Learner’s Motivation. Together, these three dimensions build the Organizational Learning Triangle as depicted in the image below.
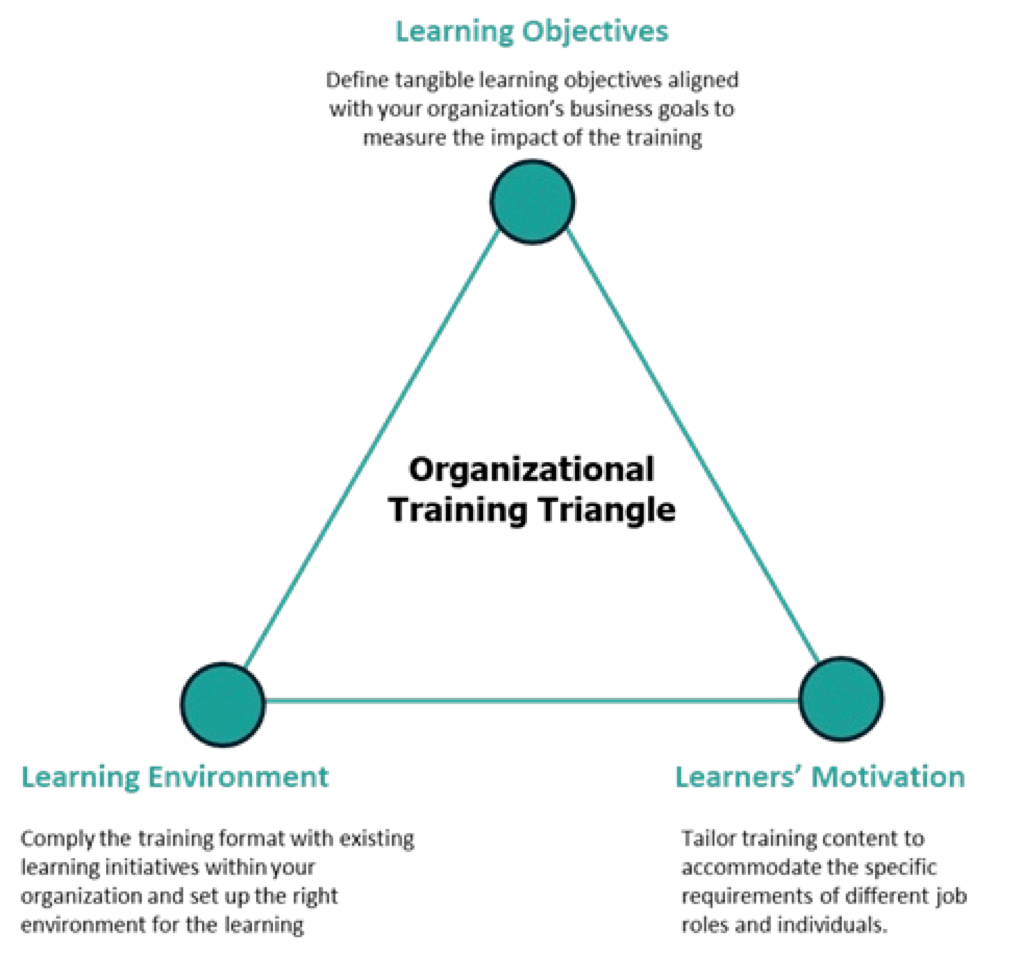
DAIN Organizational Training Triangle
When these elements are harmonized, instructional design and learning outcomes are greatly enhanced, leading to impactful results. Neglecting any of these dimensions can have severe negative effects on the learning process. When Learning Objectives are overlooked, learners may lack clear direction and purpose, resulting in confusion and disengagement. It could also happen that the training does not have the expected effect on the business if the learning objectives are not linked to business goals. Similarly, neglecting the Learning environment can lead to ineffective delivery of content, making it challenging for learners to absorb information and apply it in real-world scenarios. Furthermore, disregarding the Learner’s Motivation and the specifics of their roles can diminish enthusiasm and commitment, leading to reduced participation and retention of knowledge.
Elevating tangible learning objectives for effective training
All training initiatives should commence by establishing clear and tangible criteria for learning success. These criteria should connect with two key elements: specific, measurable business objectives, and the necessary behavioral change within the training’s target audience to attain those business goals. This also means to have evaluation and data collection mechanisms in place to track the success of the learning programs.
For data and AI literacy trainings, it is crucial to align learning objectives with the organization’s data strategy. A robust data strategy is rooted in the organization’s vision and overall business strategy. So, by linking these two elements, a strategic alignment among business objectives, data and AI initiatives, and learning strategies for data and AI literacy, is achieved.
To define tangible learning goals, start by analyzing the primary obstacles preventing the achievement of the data and AI objectives. Translate these challenges into actionable business requirements and assess the implications for re- and upskilling employees to address them effectively. Consider a scenario where a company seeks to harness data and AI to streamline operations and enhance process efficiency. But they face difficulty in developing high-impact use cases. To address this challenge, a crucial business requirement would involve increasing the number of developed use cases with significant impact across the organization’s value chain. This underscores the need to train middle management in relevant departments to adeptly identify and assess data and AI use cases using the Data Thinking approach. One metric for assessing the success of such a training could be the completion or initiation of at least one bottom-up AI use case per key function within the organization over the next year.
Optimizing the learning environment for enhanced learning experiences
Creating the right learning environment is essential for the success of any training initiative. It’s crucial that training programs are designed to fit into the overall learning strategy of the organization and align with existing learning initiatives. By fostering an environment that supports continuous learning and development, organizations can ensure that employees have the resources and support they need to acquire new skills and knowledge effectively. Additionally, providing access to modern learning technologies and resources can enhance the learning experience, making it more engaging and interactive for participants. Of course, this requires a supportive leadership that allocates sufficient resources and promotes learning opportunities.
Given the rapidly evolving nature of data and AI technologies, it’s vital that training programs are dynamic and adaptable. Organizations must create an environment that encourages experimentation and exploration, allowing employees to learn through hands-on experience and the opportunity to apply their new knowledge in their job after the training is completed. Moreover, incorporating opportunities for collaboration and knowledge sharing can enrich the learning experience and foster a culture of innovation.
A lasting impact: cultivating learners’ motivation
While convincing employees to engage in training initiatives may pose a challenge, many of them are already aware of impending disruptions by data and AI in their domain and are open to learning opportunities to maintain competitiveness. But others might hold some fears of losing their jobs or doubt their capability of learning e.g. a new programming language even though they see the importance. Employees sometimes also express skepticism about the relevance of data and AI transformation – both to the company and to their roles (source: 2022; HBR; Developing a Digital Mindset; https://hbr.org/2022/05/developing-a-digital-mindset). Therefore, the key to successful learning programs lies in the respectful treatment of employees and a transparent communication regarding the benefits and expectations of those initiatives like a new role, more decision-power, or a higher salary.
But it is not only about incentives. The key to high learner engagement lies in designing programs that resonate with employees’ preferences and are relevant for their job. Therefore, it is important to define target group personas with their specific needs, characteristics and wishes. When there are a lot of different target groups, start with the one whose training would have the biggest impact on achieving the business and learning objectives. Also, start with smaller groups and scale later. Do not try to train everybody at once with a one-fit-all solution. If a target group is too big to train at once, identify influential individuals within the target group who already possess an interest in learning about data and AI. Train them first and enlist them to advocate for data and AI programs, serving as role models for those who may be hesitant. These influencers can play an important role in pinpointing areas of employee concern and generating ideas for enhancements.
Conclusion
In the context of data and AI literacy trainings, aligning learning objectives, fostering an engaging learning environment, and igniting learner motivation are crucial for a lasting impact on data and AI transformation goals. It’s essential that such training focuses on building durable knowledge, emphasizing the shift in behavior and mindset rather than solely imparting short-term perishable knowledge of specific AI tools as there might be a new tool introduced in less than a year (source: 2020; IBM; Skills Transformation For The 2021 Workplace; https://www.ibm.com/blogs/ibm-training/skills-transformation-2021-workplace/). This also includes the opportunity to apply the newly learned skills and knowledge which might require changes in existing processes and roles, or establishing new ones. Additionally, it is crucial to establish continuous learning loops and a data and AI community within the organization so that employees have the opportunity to exchange, spread their new knowledge and keep up-to-date, building the ground for the organization to grow a data and AI literate culture.
Key Takeaways:
- Use your organization’s business strategy and data and AI goals as a base to derive impactful and tangible learning success criteria.
- Continuous leadership commitment and sponsoring is crucial as data and AI trainings take time and resources
- Focus on long-lasting behavioral change instead of short-term knowledge on trendy AI tools.
- Avoid one-fits-all learning solutions, define clear target learning personas and start with the ones that have the biggest impact on achieving your goals.
- Train a group of influential data and AI ambassadors first, that already have a certain data and AI literate mindset and are eager to learn new skills.
- Establish continuous learning loops and a data and AI community, supported by a supportive learning environment to achieve a cultural shift.