In the second blog of the series The Rise of the Data Strategist, Boyan Angelov, Senior Data Scientist, and Majella Clarke, Senior Data Strategist from DAIN Studios, present the new Data Venn and ponder – what is a data strategist? And what is the skill set needed to define and demystify the new role?
Let’s face it – Data Science blogs are awash with Venn diagrams portraying the ultimate skill set of one of the most sought-after professions this decade, but as we enter 2020, are we optimizing the management of the data talent? More specifically, are we using data capabilities and Artificial Intelligence (AI) to generate significant business impacts? That is exactly the question many businesses are currently asking themselves as the data economy evolves.
Business people know that foresight and good business strategy are essential for maintaining a competitive position. Data strategies are typically developed by business managers seeking buy-in from business leaders. On the other hand, data scientists and data engineers continue to generally have organizational alignment with IT or digital operations, even though the data strategy and vision will be developed by business people. Therefore, there is a current, and growing, need for a translational role between the data professional and business manager/leader.
In an ideal world with substantial unicorn talent, broadening the skill set required for data scientists and engineers to include business expertise, domain expertise and highly versatile communications skills, could be proposed. However, seasoned business leaders know that business decisions benefit from multi-disciplinary and cross-disciplinary insights in the form of a diverse team. The more diverse the team, the higher the collective intelligence. In the previous blog – The Rise of the Data Strategist Part I by Boyan Angelov, Boyan made a compelling case that the role of the data strategist is a highly useful role to connect data science with the business case.
In a follow-up to that blog, our Data Strategists at DAIN Studios brainstormed and designed an intersectional approach to develop and list the expertise domains, and their associated skills, that define the unique skills sets needed for a data strategist. We add to Venn diagram and data literature by proposing the following Venn for the data strategist in Figure 1 below.
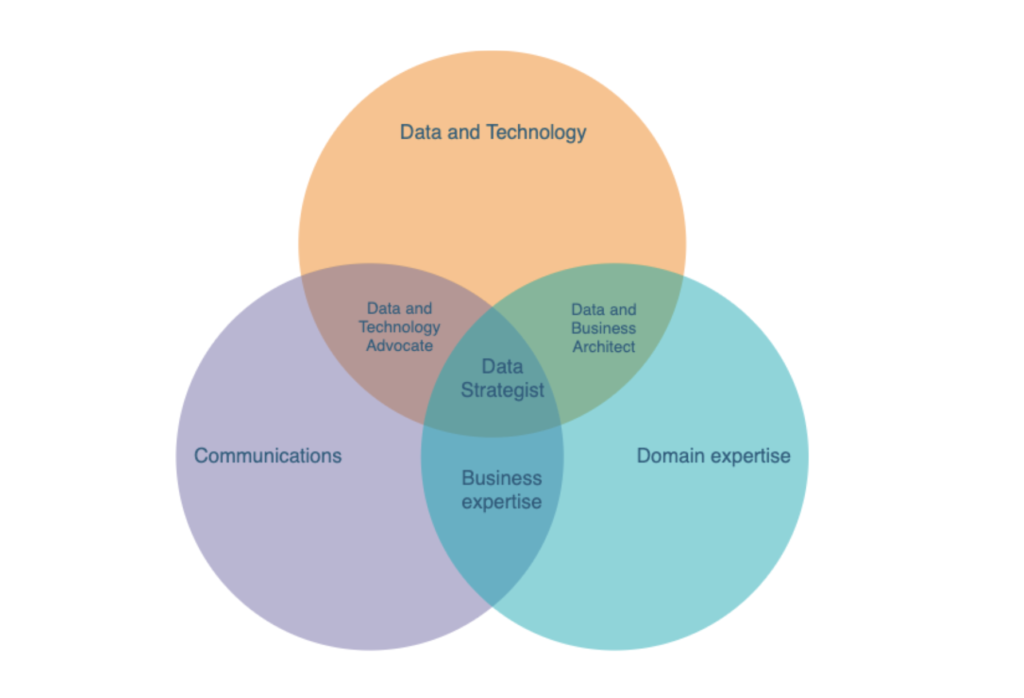
The three circles present the most important knowledge domains for a data strategist; Business, Communication, in addition to Data & Technology.
Business skills are important for a data strategist because they are required to link the data use cases with the business goals and operations. The Data & Technology knowledge domain has a clear role in the implementation of any Data and AI Strategy, however, it is important to distinguish the skills and experience on Data & Technology for a data strategist, which differ greatly from those of a data engineer. More specifically, data strategists need a good general knowledge of data infrastructure, frameworks and data management tools, but do not need to be highly experienced or skilled in engineering those frameworks and tools. Ideally, data strategists need to be aware of the broad options for current technology and data solutions, and how they can be applied to support business goals. To be able to effectively link and translate concepts on data and technology with business, strong communication skills quickly become one of the most critical skills in the translational role of the data strategist.
To further elaborate on the proposed data strategist Venn diagram, we came up with the following top skills in each of the three knowledge domains relevant for a data strategist:
Business Domain:
- Business Strategy and Use Case Knowledge: Businesses are increasingly focusing on the strategic use of data to enhance organizational performance. Therefore it is advantageous for strategists to have a library of successful (and unsuccessful) use case examples from different industries, and organizations in different stages of data maturity, to support decisions and strategic advice. The benefits should always outweigh the costs when pursuing a higher level of data and AI maturity within a company, and a data strategist will need an extensive use case arsenal to demonstrate the potential value of a data investment.
- Cross Functional and Cross Domain Business Translation Skills: End-to-End (E2E) solutions require cross functional and cross domain knowledge and skills to be able to conceptualize and translate “the big picture” to teams. Data strategists need to be able to see how different business domains and functions within an organization can work together to solve problems and innovate new products and services. Such knowledge requires application when, for example, siloed domain experts could be unaware of the data and solutions other siloed domain experts are using, often missing out on valuable business insights with reusable datasets.
- Governance and Data Protocols: Recent legislation and regulations to protect personal data and recognize the importance of cyber security require new protocols that need to be managed within business functions. In addition, automation and recent technology developments (e.g. 5G, Cloud etc) have created new ways of working. Data strategists need to be aware of their organizational data protocols as well as the jurisdictional legalities that protect and safeguard data. Poor data governance can have strong potential implications to the overall business strategy.
- Project Management: project management is an essential business skill. For a data strategist, knowledge of different project management practices, from scrum to waterfall, will be useful, as many advanced analytics projects are initially structured through shorter project / pilot approaches before they are rolled-out into enterprise wide actions.
- Creativity and Complex Problem Solving: Computational thinking and data science change the way we think about problems and develop business solutions. Familiarity with methods such as design thinking, brainstorming, reduction and abstraction, for example, can help leverage Artificial Intelligence (AI) for innovation and gain deep insights to problems using data science.
- Strategic Human Resource Management for Data Roles: It is very important for a strategist to understand what the different roles in the data domain are (i.e. scientists, engineers, business intelligence developers and others), how they work together and what compositions of data experts are needed for different use cases.
- Foresight: often termed as one of the most important business and leadership skills of the 21st century, foresight is about planning for the future using different strategic methods to scan and source evolving pathways. Digital transformation and technology have created on one hand a business environment that is undergoing rapid changes. Large amounts of automated data with machine learning also provide new ways to deploy predictive analytics to gain future insights.
Data & Technology Domain:
- Data Asset Management: As with any business strategist, understanding and growing the value of business assets is a prerequisite for performance. Data Asset Management (DAM) is a key skill for data strategists because the outcomes, quality of recommendations and advice of data strategists is increasingly connected with the quality of the data. Therefore data strategists need to be aware of the best practices for collecting, curating and managing the value of data within an organization. In addition, it is important to know what methods are suitable for which types of data (i.e. computer vision vs. text, data 360). For example, Deep Learning methods are often more useful when working with large amounts of unstructured data.
- General Knowledge of Machine-Learning Methods: While it is certainly not necessary for a strategist to be able to build and deploy Machine Learning (ML) models, it is nevertheless essential that they have a good understanding of the different techniques currently available. For example, supervised vs. unsupervised methods, regression vs classification and deep learning. Understanding the essential ML methods and the relevant potential business use cases and analytical insights for which they can be deployed is of strategic business importance for also maintaining an effective algorithm portfolio.
- Data Engineering Awareness: Knowing the puzzle pieces for setting up viable data infrastructure and architecture are essential to support the implementation of a data, AI and/or digital transformation strategy. To this point, the questions of database selection, cloud provider and various open source technologies, should be made to fit the business strategy by the data strategist. Consideration of performance of the architecture and technology versus the cost efficiency are key factors in the decision making process.
- Data Ethics: Now that machine learning systems are being deployed in more critical industries (such as healthcare and government, among others), it is of utmost importance that ethics permeate every aspect of the work. Successful machine learning systems have generalizable results. For this to work, the data has to be representative of the task at hand. Additionally, those data must be free of bias – here methods from an evolving field termed eXplainable Artificial Intelligence (xAI) can be applied within different parts of the system (i.e. in data collection, model training, deployed model debugging).
Communication Domain:
- Data Visualization: a picture says a thousand words and can convey the concept using vast amounts of data very quickly. Data visualization is a powerful skill that can persuade and awe business leaders and managers, and drive change.
- Listening skills: listening is the other side of the translational role. Listening is becoming important because it is crucial to productivity and managing the emotions and reactions of teams and people.
- Presentation skills: With all the data-driven insights, delivery is important when communicating complex and technical concepts to business people that have different skill sets. Presentation skills are where the translational role of the data strategist culminates. For instance, a data strategist discussing analytics with a data scientist will use a very different translational language in the delivery and discussion of results compared with the context when discussing the same results with the CEO / C-suite.
- Coaching and Mentoring skills: The path to advanced analytics can be long and weary, and coaching managers through the barriers and resistance, as well as giving managers and their teams the courage and confidence to implement the data strategy roadmap are highly appreciated and valued.
- Change Management: is perhaps the most underestimated skill in the implementation of a data strategy or any digital transformation process. Once the digital transformation process starts within an organization and the Return on Investment of pursuing a high level of analytics maturity is understood by business leaders, changes need to be accompanied by timely and appropriate communication. A good change manager can gain trust in a constantly changing, abstract environment, and create a smooth change process that business leaders commit to for the long run. Change management is the skill that data strategists need to ensure an efficient implementation of the data strategy.
The need for technical generalist business people is still growing. The skills that support the role requirements of a data strategist bring together the domains of Technology & Data, Communications and Business. On the bilateral data strategy domain intersections, we have Data and Business Architect that are highly versed in cross-domain Data & Technology solutions, with knowledge of cross-functional business processes and objectives; Business Domain Experts are leaders that are confluent in communications and business operations and administration. Data and Technology Advocates are highly knowledgeable and experienced in the Technology & Data domain, with well-developed translational skills that can communicate complex data and technology concepts at the appropriate level of engagement. The data strategist unicorn, we argue, operates within all three domains to guide leaders and organizations towards the implementation of a highly data centric business strategy that capitalizes on and monetizes its data assets.