To date, many companies are investing in Data and Artificial Intelligence (AI). As the terminology varies, the activities may be called Artificial Intelligence, Advanced Analytics, Data Science, or Machine Learning, but the goals are the same across companies: Increase revenues and efficiency in current business, and develop new data-enabled offerings. It is well understood that to stay competitive in the digital economy, the company’s internal processes and products need to be smart – and smartness comes from Data and AI. Successful Digital transformation is not possible without Data and AI.
Over the past four years, DAIN Studios has been involved in more than 50 Data and AI initiatives in different companies and industries in Finland, Germany, Austria, Switzerland, and the Netherlands. We have defined Data and AI strategies, evaluated AI execution projects, and advised companies on topics such as data governance, organization, and operating model. We have also built cloud infrastructures, engineered data, and developed scalable machine-learning models. We have advised dozens of business leaders on how to become data driven and use AI to their benefit. This article reviews some of the findings that we have made and proposes best practices for going forward.
The reality is that there are no shortcuts. Overcoming those matters calls for strong determination and persistence from the company leadership. It means bringing Data and AI into the core of all aspects of decision making – from strategy to operations, supported by Key Performance Indicators that align data-driven decision making.
Based on our experience, business leaders need to be highly involved in all aspects of the execution of data and AI strategies and the capabilities that the supporting initiatives involve. We observe that fully committed leadership has been one of the common denominators for success in digital transformation and becoming a data-driven company.
Business leaders are to put their efforts into creating an effective company environment for Data and AI. That means setting business goals, hiring the right people, educating the workforce, committing to investments, and implementing an effective operating model and organization for Data and AI. This is best done by setting clear goals and incentives for the organization and following up on them.
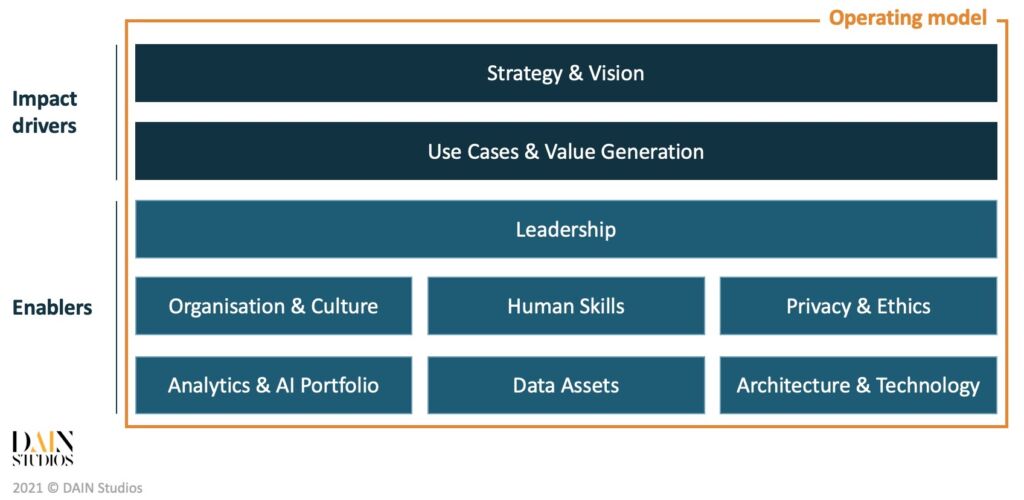
In the next section, we go deeper into the topics that should be accounted for when defining and executing a Data and AI Strategy.
Setting the Data and AI Vision
The premise for successful Data and AI Strategy is to know your business goals. What are your must-win battles? Where do you need to succeed in the future? Data and AI will help you make more informed decisions, obtain information faster, automate processes, and enable delivery faster than a human mind – but it will not construct or replace the lack of business vision and ideas.
AI priorities are derived from business priorities. Since Data and AI will make different contributions in different areas, when assessing where to focus a company’s Data and AI efforts, one should consider the business case for each business area as well as AI’s relative importance to the case.
Calculating business cases for cost-saving cases is easier than for new business, though product development can eventually results in large impactful outcomes. Early wins are important to communicate to obtain buy-in from the organization, as well as increase general understanding on demonstrated AI benefits. Gaining support and buy-in for a Data and AI vision is equally important. Strategizing along these lines is a good exercise for understanding the big picture.
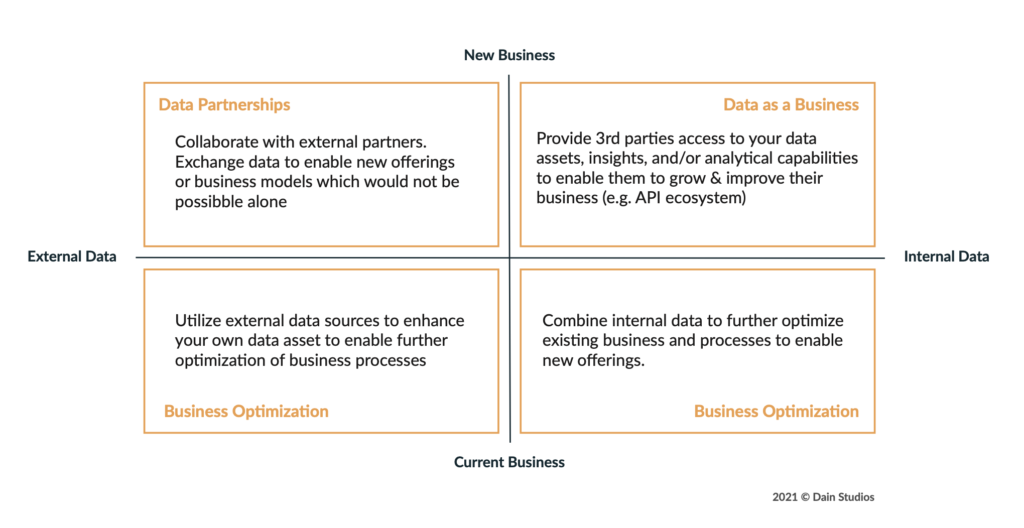
Data Asset Management / Data Governance
The availability of high-quality data is the foundation for successful, productized AI. Data can be called an Asset if it is structured according to the FAIR principles (Findable – Accessible – Interoperable – Reusable) as suggested by the EU Horizon 2020 Program (2016). The principle of “Build Once – Use Many” is pivotal for maximizing the value of Data Assets. The goal of a productized Data Asset is to support all use cases.
When building your company’s Data Asset, start with the data needed for the prioritized business opportunities/use cases.
One good practical way to start building a Data Asset is to take stock of the current data assessing how “FAIR” the data is. The process is called Data Due Diligence or Data Inventory.
A Data Due Diligence responds to questions such as the following:
- What data exists?
- Where does it reside?
- How can it be accessed?
- What is its quality?
- Can it be linked to other data?
- How much effort does its retrieval take?
- Do we miss some obvious data sources (considering the use cases)?
Once the current state of the Data Asset has been identified, a roadmap for its development can be made.
Solution Architecture and Technology
One of the first tasks after the definition of the Business & AI vision and Data Due Diligence is to have an experienced Data and Solution Architect take a critical look into the current technical architecture and define the target architecture and its development roadmap. This task, too, should follow the end-to-end use case logic accounting for data collection from operating systems (e.g. CRM, ERP), data warehouses, cloud environments, analytical environments, and business-interfacing systems. Automated Machine-Learning/AI solutions need to be linked back to operative systems meaning that operative systems need to be an integral part of the data and solution architecture. For example, to use your Consumer Data Asset (including individual micro-segments, next-best offers, and other consumer scores) in real time as part of a modern, omnichannel Marketing Automation system, you need to set up end-to-end architecture.
Managing the transition from traditional IT systems into the digital world is often a lengthy process. While Automation and AI will eventually drive costs down, during the transition time, costs are likely to increase as new and old solutions live side by side.
Data Protection and Privacy
Data Protection and Privacy is of key interest to consumers and those with access to consumer data. When using data and ensuring compliance with the privacy legislation, it is critical to write the privacy policies according to the desired state of the AI use cases, not the current cases. The point is to avoid a situation where your privacy policy allows you to use your data only for reporting purposes while you want to develop hyper-targeted, personalized AI models.
A good team for setting up the company-wide privacy policies consists of a combination of Business Owners, Privacy Lawyers, and AI Strategists (or Data Scientists). AI Strategists with a technical background will help translate the business use cases into Data and AI requirements and discuss the interpretations of different options (including User Experience) with Privacy Lawyers.
Human Skills
The Data and AI journey requires new roles in an organization. A good start is to hire a Chief Data and AI Officer with experience in business, data, data science, and technology to hire the talent and set up the teams. In addition to subject-matter expertise, this person should have excellent leadership and communication skills as they need to communicate effectively with different levels of people in the organization. While the exact role terminology varies, Data and AI roles are needed for four different levels of business processes:
- Business Units (P/L) and Business Functions (e.g. Sales, Marketing, Finance)
- Data Science (and Business Intelligence)
- Data Asset Management
- Data Platforms and Technical Solutions
The business use cases come from the business level (#1). In addition to actual business people, the role of the AI Strategist resides here. The AI Strategist translates the business vision and goals into Data and AI requirements, oversees project execution, and ensures that project outcomes are taken into use by business processes. Most companies do not have this role, but we see it as one of the most critical roles in the successful execution of Data and AI projects.
In addition to AI Strategists, business leaders themselves also need to have an understanding of the opportunities of Data and AI in order to drive the topic forward and integrate the AI outcomes into their respective business processes. Data Scientists (#2) come in various forms, with different backgrounds. It makes sense to have a Data Science team with different types of educational backgrounds.
We recommend recruiting a senior Data Scientist or an AI Strategist as the first hire and let them build a balanced team consisting of experienced people and promising young talent. A common mistake is to hire only Data Scientists and not fill the technical roles such as Data Engineers and Data Architects (#3) or Platform Engineers and Solution Architects (#4).
Data and AI Organization
The optimal Data and AI organization structure depends on the overall company size and organization, culture, the level of AI maturity, and the type of Data/AI tasks.
Generally, to get things going, establishing a Center of Excellence (CoE) brings focus into the topic. Depending on where the CoE sits in a company, it will be responsible for different areas. The CoE may consist of Data Science & BI teams only while the technical teams (Data Engineering, Platforms) reside in IT. In our experience, most companies will benefit from a common technical infrastructure and Data Asset, as well as some type of a centralized Data Science team, which solves the most difficult use cases and creates a scalable AI portfolio for the use of all business units and functions.
The AI Strategists should optimally sit within business units to drive the AI use cases forward, but in the beginning, they can also reside with the Data Science teams and help business from there.
Sometimes, to make starting easy, it makes sense to introduce a company-wide AI Program to drive the Data and AI agenda forward – with the premise that the program exists for 2-3 years and will then be dissolved. A program’s benefit is that you do not need to make line-organizational decisions in the early phase, but will learn over time what type of team structure works for your organization.
Operating Model
A closely related topic to Data and AI Organization is the Operating Model between different business units. Prioritized business use cases should drive the Data and AI development. In order to have the Data Experts work on the most important use cases, the data and AI use cases should be on the leadership team’s agenda and steering groups should be formed as needed.
For the first years of the CoE, Data and AI development budgets should be centralized. Budgets drive prioritization, and without a centralized budget, Data and AI activities will not scale up. It is important to remember that it is not only about the data experts. Business processes and business people are fundamentally impacted by the utilization of Data and AI.
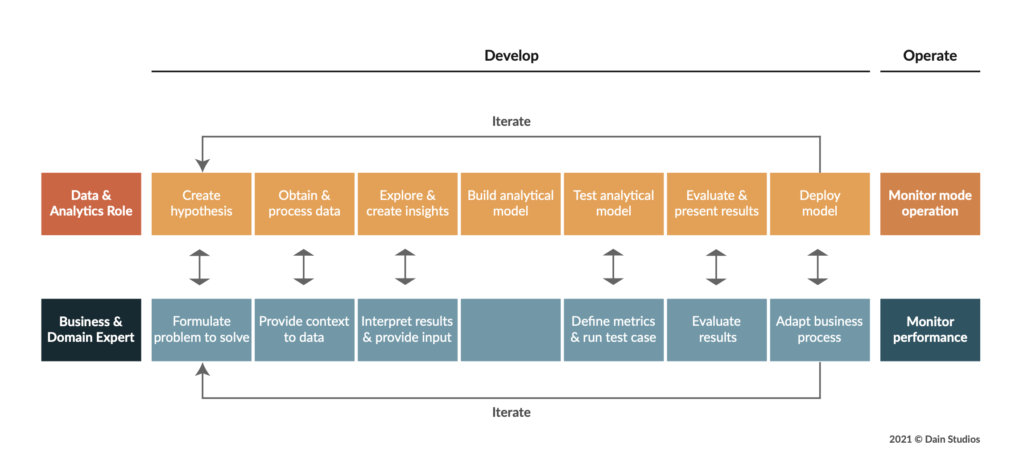
Close collaboration between the data and business functions secures tangible and sustainable results.The parallel between technical and business development processes is illustrated below.
Data Science and Machine Learning/AI Algorithms
Like the Data Asset, algorithms can also be treated as an Algorithm Asset. That means that over time, the portfolio of machine-learning/AI algorithms will become FAIR. Every new analytical modeling exercise does not need to start from scratch but builds on top of tested code. This will make the Data Science team more efficient over time. Like software coding teams, it requires the Data Science team to use common code repositories and standards.
It is also important to establish maintenance processes for the Data and Algorithm Assets. If maintenance processes remain undeployed, development teams remain in a state of stagnation as their efforts go into keeping the current Assets in production. By applying maintenance processes to data and algorithm portfolios, new solutions can be discovered and developed.
Final Words
To summarize, the following steps are needed to execute Data and AI successfully:
- Translate your business and digital strategy into your Data and AI vision and strategy highlighting the biggest opportunity areas optimizing your current business as well as new innovative businesses utilizing AI and Data;
- Identify the business processes (product development, production, sales & marketing, supply chain, pricing, HR, finance, etc.) where you want to use Data and AI;
- Understand the current state of your Data and AI capabilities;
- Describe the target state for your business processes once Data and AI capabilities have been deployed;
- Define new data-driven business and product ideas;
- Define your execution roadmap, including investments;
- Execute the first Data and AI use cases by creating your AI Playbook, aiming at production readiness;
- Automate and scale up operations.
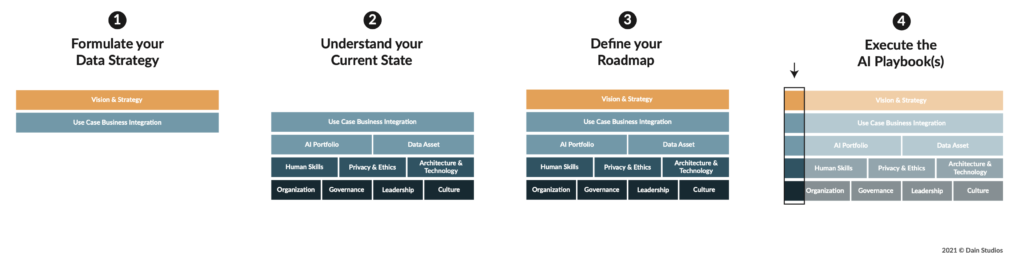
Sometimes people think that the highest level of an AI-driven company is when all decision-making and business processes are done by automated algorithms. That is, however, a misconception. Automation and AI do not make smart business decisions by themselves. The highest level of AI maturity is when the whole company goes into one direction, silos are dissolved, and Data and AI are used by everyone as part of their daily business. Everything that can be automated will be automated, and humans need to ensure that automation is done in a smart way.